Overview: What are Genetic Algorithms?
Genetic Algorithms (GAs) are a class of optimization and search algorithms inspired by the principles of natural selection and genetics. GAs are widely used in artificial intelligence (AI), machine learning, engineering, and operations research to solve complex optimization problems where traditional techniques struggle.
Contributions to the AI Field
- GAs provide an effective heuristic search method for solving non-linear, multi-dimensional, and large-scale optimization problems.
- They contribute to fields like machine learning, feature selection, robotics, and game AI.
- GAs help in evolving neural networks and automating AI decision-making.
This article provides an in-depth introduction to Genetic Algorithms, covering their historical development, technical details, and real-world applications. The goal is to help readers understand GAs as a stepping stone to more advanced AI topics such as Game Theory, Reinforcement Learning, and Evolutionary Computing.
1. Development History of Genetic Algorithms
Origins and Key Figures
Genetic Algorithms are inspired by Charles Darwin’s theory of natural selection and evolution (1859). However, their computational application began in the 20th century.
John Holland (Father of GAs) – 1960s
- Developed the first formal GA models at the University of Michigan.
- Introduced key genetic operators: selection, crossover, and mutation.
- Published “Adaptation in Natural and Artificial Systems” (1975).
David E. Goldberg – 1989
- Expanded GA research into engineering and industrial applications.
- Published “Genetic Algorithms in Search, Optimization & Machine Learning”, a foundational text in the field.
Kenneth De Jong (1975)
- Conducted early experimental studies demonstrating GAs’ efficiency compared to conventional optimization methods.
John Koza – 1990s
- Developed Genetic Programming (GP), extending GAs to evolve complete computer programs.
Key Studies & Achievements
- Holland’s Schema Theorem (1975) laid the mathematical foundation for GA search efficiency.
- Goldberg’s Research (1989) applied GAs to real-world control and scheduling problems.
- NASA used GAs to evolve efficient satellite antenna designs, surpassing human-engineered solutions.
2. How Genetic Algorithms Work: Technical Description
Genetic Algorithms mimic biological evolution through iterative cycles of selection, crossover, and mutation to refine candidate solutions over time.

Basic GA Workflow
- Initialization: Generate a random population of potential solutions.
- Fitness Evaluation: Assess the quality of each solution using a fitness function.
- Selection: Choose the best solutions for reproduction.
- Crossover (Recombination): Combine solutions to create new offspring.
- Mutation: Introduce random variations for diversity.
- Replacement: Replace old solutions with newly generated ones.
- Termination: Stop when an optimal or near-optimal solution is found.
Genetic Operators
Selection Methods
- Roulette Wheel Selection – Probability-based selection where fitter solutions have a higher chance.
- Tournament Selection – Randomly selects a subset and picks the best solution.
- Elitism – Ensures top-performing solutions persist across generations.
Crossover Techniques
- Single-Point Crossover – Splits parent solutions at one point and swaps segments.
- Uniform Crossover – Randomly mixes genes from parents.
Mutation Strategies
- Bit Flip Mutation – Randomly flips bits in binary encoding.
- Gaussian Mutation – Adjusts numerical values slightly.
Encoding Strategies
- Binary Encoding – Solutions represented as binary strings (e.g.,
101011
). - Permutation Encoding – Used for ordering problems like the Traveling Salesman Problem (TSP).
- Real-Valued Encoding – Used for continuous parameter optimization.
3. Real-World Applications & Case Studies
Example: Traveling Salesman Problem (TSP)
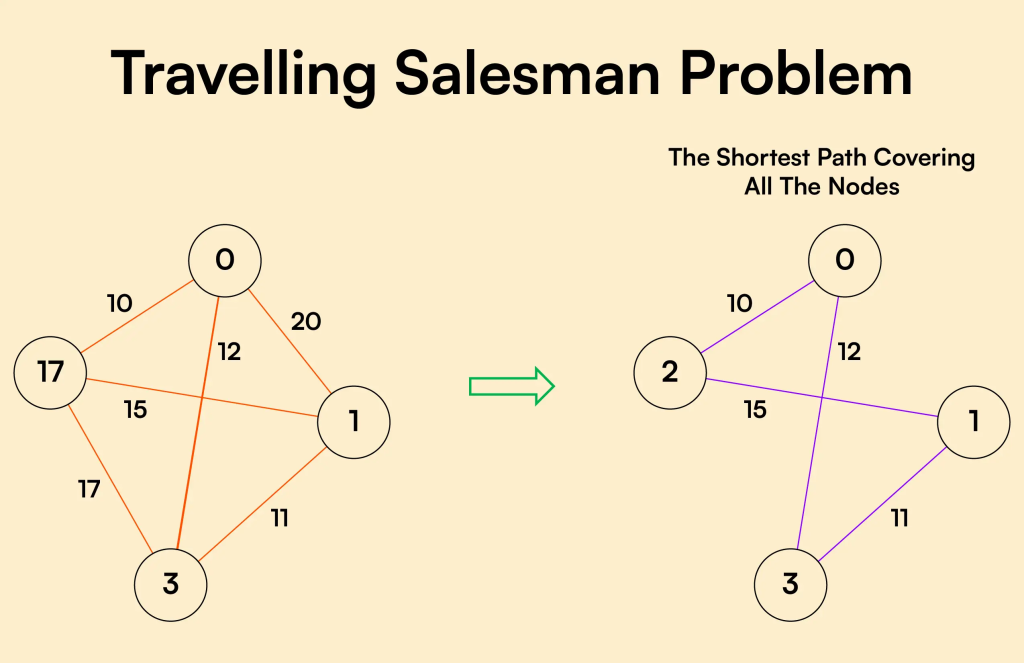
Problem: A salesman must visit N cities while minimizing the total travel distance.
GA Approach:
- Encoding: A route is represented as a sequence of cities.
- Fitness Function: The total distance of the route.
- Selection, Crossover & Mutation: Combine and tweak routes to find better solutions.
GAs outperform brute-force methods for large TSP instances.
Case Study: NASA’s GA-Based Antenna Design
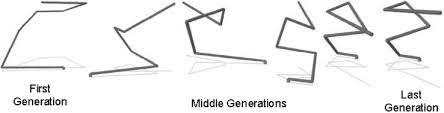
Problem: NASA needed an antenna design with optimal signal strength for space missions.
GA Solution:
- GAs evolved antenna shapes over multiple generations.
- The final design outperformed traditional human-engineered solutions.
This highlights GAs’ ability to innovate beyond human intuition.
Application in AI & Machine Learning: Feature Selection
Problem: Selecting the best features for a machine learning model.
GA Solution:
- Encoding: Each solution represents a subset of features.
- Fitness Function: The ML model’s accuracy on training data.
- Crossover & Mutation: Generates better feature subsets.
GAs improve accuracy and reduce model complexity.
GA in Game AI & Robotics
- Game AI: Evolving NPC behaviors for dynamic game environments.
- Robotics: Optimizing robot motion planning and control systems.
Conclusion
Genetic Algorithms have proven to be a powerful optimization tool in AI, machine learning, and engineering. Their ability to efficiently search for optimal solutions makes them fundamental in real-world applications such as logistics, scheduling, robotics, and AI development.
(Study) Dive deeper into Genetic Algorithm
- Course (text) (tutorialspoint) genetic_algorithms
- Course (~ 6 hours) (youtube) 9.1: Genetic Algorithm:
(Coding, Libraries) Genetic Algorithm frameworks
- Java MOEA framework: moeaframework.org
- Python PyGAD: PyGAD – Python Genetic Algorithm! — PyGAD 3.3.1 documentation